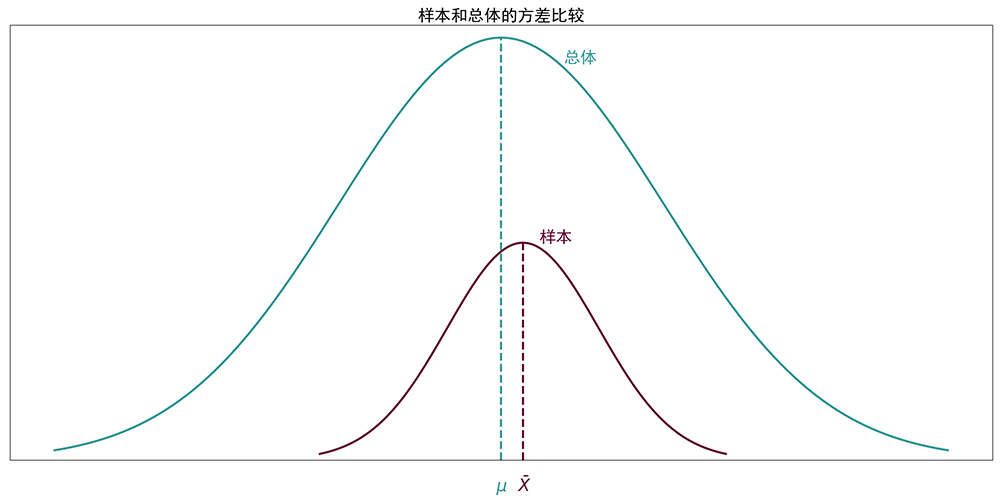
绘制所用的Python代码如下:
import matplotlib.pyplot as plt
from scipy import linspace, sqrt
from scipy.special import erf
import numpy as np
color = '#339999'
color2 = '#660033'
def pdf(x, mu=0, sigma=1):
"""
Calculates the normal distribution's probability density
function (PDF).
"""
term1 = 1.0 / (sqrt(2 * np.pi) * sigma)
term2 = np.exp(-0.5 * ((x - mu) / sigma) ** 2)
return term1 * term2
def cdf(_x):
return (1 + erf(_x / sqrt(2))) / 2
def skew(_x, e, _w, a, z=100):
t = (_x - e) / _w
return z / _w * pdf(t) * cdf(a * t)
plt.figure(figsize=(20, 10))
plt.rcParams['font.sans-serif'] = ['SimHei']
plt.rcParams['axes.unicode_minus'] = False
n = 129
w = 2.5
y_text_offset = -0.5
y_title_offset = 8.1
fontsize = 24
middle_x = 0
x = linspace(middle_x - w * 3.3, middle_x + w * 3.3, n)
p = skew(x, middle_x, 3, 0)
plt.plot(x, p, 'r-', color=color, linewidth=3.0)
middle_index = list(p).index(max(p))
plt.text(x[middle_index + 12] - 0.4, max(p)-0.4, '总体', fontsize=fontsize, color=color)
plt.plot([x[middle_index], x[middle_index]], [0, p[middle_index]], '--', color=color,
linewidth=3.0)
plt.text(x[middle_index] - 0.1, y_text_offset, '$\mu$', fontsize=fontsize, color=color)
plt.ylim([0, max(p) + 0.2])
middle_x = middle_x + 0.4
x = linspace(middle_x - w * 1.5, middle_x + w * 1.5, n)
p = skew(x, middle_x, 1.4, 0, 24)
plt.plot(x, p, 'r-', color=color2, linewidth=3.0)
plt.text(x[middle_index + 12] - 0.4, max(p), '样本', fontsize=fontsize, color=color2)
plt.plot([x[middle_index], x[middle_index]], [0, p[middle_index]], '--', color=color2,
linewidth=3.0)
plt.text(x[middle_index] - 0.1, y_text_offset, r'$\barX$', fontsize=fontsize, color=color2)
print([min(x), max(x)])
plt.plot([min(x), max(x)], [y_text_offset, y_text_offset], 'r-', color=color2,
linewidth=3.0)
plt.title('样本和总体的方差比较', fontsize=fontsize)
plt.yticks([])
plt.xticks([])
plt.show()